No, Maybe and Close Enough! Probabilistic Data Structures with Python and Redis
Session Type | Pre-Recorded |
---|---|
Start time | 16:45 |
End time | 17:15 |
Countdown link | Open timer |
Being right all the time isn't necessarily the best idea. This talk examines how to count distinct items from a firehose of data, how to determine if we've seen a given item before, and why absolute accuracy may be impractical when doing so.
Probabilistic data structures trade accuracy for approximate results, speed and economy of resources. They provide fast, scalable solutions to problems such as counting likes on social media posts, or determining which articles on a website a user has previously read.
I'll introduce the Hyperloglog and Bloom Filter, explain how they work at a high level, and demonstrate different ways in which each can be leveraged in Python.
This talk is aimed at Python developers at any experience level who face challenges associated with processing large data sets. It is also for anyone wanting to learn about what problems probabilistic data structures solve, when to use them and different ways in which they can be added to a Python application. Audience members should be familiar with Python syntax and the Pip package installer. This talk will cover basic mathematical set theory, but prior knowledge of this is not required. After watching this talk, the audience should expect to know what Hyperloglogs and Bloom Filters are, when to use them, what trade offs are involved, and where implementations of each are available.
0-10 minutes:
- Counting things, and remembering if we've seen them before... how hard can it be?
- Using Sets for absolute accuracy.
- Issues with using Sets...
- Let's use a database instead?
10-15 minutes:
- The case for approximation. Do we really need absolute accuracy?
- Introducing Probabilistic Data Structures and hashing.
15-25 minutes:
- Adding Hyperloglog and Bloom Filters to a Python application with pyprobables.
- Using Redis as a probabilistic data structures server with a Python application.
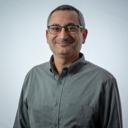
Simon Prickett is a senior software developer and technical trainer. He enjoys projects that fuse hardware and software, especially with Arduino and Raspberry Pi. Simon brings experience gained from living and working on three different continents in industries including banking, logistics, IoT and Software as a Service platform development.
Find him online at https://simonprickett.dev